
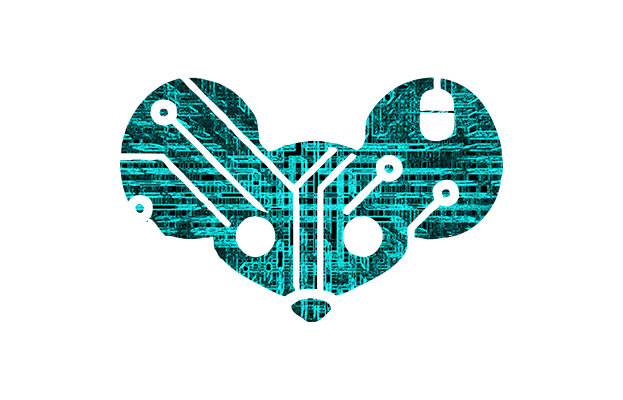
I think it’s been about a year? IIRC Intel only started using TSMC for their processors with Meteor Lake, which was released in late 2023.
I believe their discrete GPUs have been manufactured at TSMC for longer than that, though.
I think it’s been about a year? IIRC Intel only started using TSMC for their processors with Meteor Lake, which was released in late 2023.
I believe their discrete GPUs have been manufactured at TSMC for longer than that, though.
I use a lot of AI/DL-based tools in my personal life and hobbies. As a photographer, DL-based denoising means I can get better photos, especially in low light. DL-based deconvolution tools help to sharpen my astrophotos as well. The deep learning based subject tracking on my camera also helps me get more in focus shots of wildlife. As a birder, tools like Merlin BirdID’s audio recognition and image classification methods are helpful when I encounter a bird I don’t yet know how to identify.
I don’t typically use GenAI (LLMs, diffusion models) in my personal life, but Microsoft Copilot does help me write visualization scripts for my research. I can never remember the right methods for visualization libraries in Python, and Copilot/ChatGPT do a pretty good job at that.
There is no “artificial intelligence” so there are no use cases. None of the examples in this thread show any actual intelligence.
There certainly is (narrow) artificial intelligence. The examples in this thread are almost all deep learning models, which fall under ML, which in turn falls under the field of AI. They’re all artificial intelligence approaches, even if they aren’t artificial general intelligence, which more closely aligns with what a layperson thinks of when they say AI.
The problem with your characterization (showing “actual intelligence”) is that it’s super subjective. Historically, being able to play Go and to a lesser extent Chess at a professional level was considered to require intelligence. Now that algorithms can play these games, folks (even those in the field) no longer think they require intelligence and shift the goal posts. The same was said about many CV tasks like classification and segmentation until modern methods became very accurate.
GPU and overall firmware support is always better on x86 systems, so makes sense that you switched to that for your application. Performance is also usually better if you don’t explicitly need low power. In my use case I use the Orange Pi 5 Plus for running an astrophotography rig, so I needed something that was low power, could run Linux easily, had USB 3, reasonable single core performance, and preferably had the possibility of an upgradable A key WiFi card and a full speed NVMe E key slot for storage (preferably PCIe 3.0x4 or better). Having hardware serial ports was a plus too. x86 boxes would’ve been preferable but a lot of the cheaper stuff are older Intel mini PCs which have pretty poor battery life, and the newer power efficient stuff (N100 based) is more expensive and the cheaper ones I found tended to have onboard soldered WiFi cards unfortunately. Accordingly the Orange Pi 5 Plus ended up being my cheapest option that ticked all my boxes. If only software support was as good as x86!
Interesting to hear about the NPU. I work in CV and I’ve wondered how usable the NPU was. How did you integrate deep learning models with it? I presume there’s some conversion from runtime frameworks like ONNX to the NPU’s toolkit, but I’d love to learn more.
I’m also aware that Collabora has gotten the NPU drivers upstreamed, but I don’t know how NPUs are traditionally interfaced with on Linux.
A lot of the cheap tablet SoC vendors like Rockchip (whose SoCs end up in low cost SBCs) really only do the bare minimum when it comes to proper linux support. There’s usually next to no effort to upstreaming their patches so oftentimes you’re stuck on their vendor kernel. Luckily for the RK3588(S), Collabora has done a considerable amount of work on supporting the SoC and its peripherals upstream. I run my Orange Pi 5 Plus (RK3588) on a mainline kernel and it works for my needs.
This practice is a lot easier to defend for a low cost SoC compared to something as expensive as a Snapdragon Elite though…
Yep, and for good reason honestly. I work in CV and while I don’t work on autonomous vehicles, many of the folks I know have previously worked at companies or research institutes on these kinds of problems and all of them agree that in a scenario like this, you should treat the state of the vehicle as compromised and go into an error/shutdown mode.
Nobody wants to give their vehicle an override that can potentially harm the safety of those inside it or around it, and practically speaking there aren’t many options that guarantee safety other than this.
Yeah I think Lemmy would actually work pretty reasonably. It reminds me of how lots of software and projects have Reddit communities. I agree that being able to share 1 account over many services, and especially not having to pay for infrastructure is something that drives discord use over forum-based platforms.
Personally, I’d prefer that projects use forums for community discussions rather than realtime chat platforms like Discord or Matrix. I think the bigger problem of projects using Discord is not that it’s closed source, but rather that it makes it difficult to search (since no indexing by search engines) and the format deprioritizes having discussion on a topic over a long period of time. Since Matrix is also intended for chat, it has these same issues (though at least you can preview a room without making an account).
Afaik the StarFive SOCs used in SBCs are a lot slower than current ARM offerings. Part of that might be because software support is worse, so maybe compilers and related tooling aren’t yet optimized for them?
Hopefully development on these continues to improve though. The biggest nail in the coffin for Pi alternatives has been software support.
As of right now VLC also doesn’t properly support Wayland, but MPV does. It’s a great piece of software!
Agree on the sentiment about VLC though, having an open source project demonstrate what is possible and stand the test of time definitely paves the way for future work and improvements.
I’m a researcher in ML and that’s not the definition that I’ve heard. Normally the way I’ve seen AI defined is any computational method with the ability to complete tasks that are thought to require intelligence.
This definition admittedly sucks. It’s very vague, and it comes with the problem that the bar for requiring intelligence shifts every time the field solves something new. We sort of go “well, given these relatively simple methods could solve it, I guess it couldn’t have really required intelligence.”
The definition you listed is generally more in line with AGI, which is what people likely think of when they hear the term AI.
I believe this is the referenced article:
I think what they mean is that ML models generally don’t directly store their training data, but that they instead use it to form a compressed latent space. Some elements of the training data may be perfectly recoverable from the latent space, but most won’t be. It’s not very surprising as a result that you can get it to reproduce copyrighted material word for word.
Not sure what other people were claiming, but normally the point being made is that it’s not possible for a network to memorize a significant portion of its training data. It can definitely memorize significant portions of individual copyrighted works (like shown here), but the whole dataset is far too large compared to the model’s weights to be memorized.
The big thing you get with frameworks is super simple repairability. This means service manuals, parts availability, easy access to components like the battery, RAM, ssd, etc. Customizable ports are also a nice feature. You can even upgrade the motherboard later down the line instead of buying a whole new laptop.
I haven’t read the article myself, but it’s worth noting that in CS as a whole and especially ML/CV/NLP, selective conferences are generally seen as the gold standard for publications compared to journals. The top conferences include NeurIPS, ICLR, ICML, CVPR for CV and EMNLP for NLP.
It looks like the journal in question is a physical sciences journal as well, though I haven’t looked much into it.
I’m curious what field you’re in. I’m in computer vision and ML and most conferences have clauses saying not to use ChatGPT or other LLM tools. However, most of the folks I work with see no issue with using LLMs to assist in sentence structure, wording, etc, but they generally don’t approve of using LLMs to write accuracy critical sections (such as background, or results) outside of things like rewording.
I suspect part of the reason conferences are hesitant to allow LLM usage has to do with copyright, since that’s still somewhat of a gray area in the US AFAIK.
Also one very important aspect of this is that it must be possible to backpropagate the discriminator. If you just have access to inference on a detector of some kind but not the model weights and architecture itself, you won’t be able to perform backpropagation and therefore can’t generate gradients to update your generator’s weights.
That said, yes, GANs have somewhat fallen out of favor due to their relatively poor sample diversity compared to diffusion models.
For reference, ICML is one of the most prestigious machine learning conferences alongside ICLR and NeurIPS.
Yeah we used to joke that if you wanted to sell a car with high-resolution LiDAR, the LiDAR sensor would cost as much as the car. I think others in this thread are conflating the price of other forms of LiDAR (usually sparse and low resolution, like that on 3D printers) with that of dense, high resolution LiDAR. However, the cost has definitely still come down.
I agree that perception models aren’t great at this task yet. IMO monodepth never produces reliable 3D point clouds, even though the depth maps and metrics look reasonable. MVS does better but is still prone to errors. I do wonder if any companies are considering depth completion with sparse LiDAR instead. The papers I’ve seen on this topic usually produce much more convincing pointclouds.